Web-scale Hybrid Explainable Learning
Day 2 | 11:00 – 12:00 | Main Hall
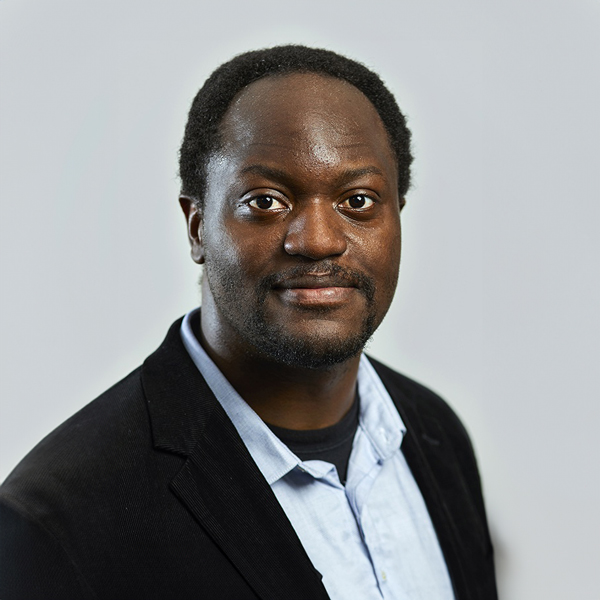
Prof. Dr. Axel-Cyrille Ngonga Ngomo
Paderborn University
Abstract
Foundation models are a key component of a growing number of modern applications. In particular, modern large language models achieve close-to-human performance in language generation. However, by virtue of being statistical models, they are often unable to stay true to the assertional knowledge in their training data. We address this challenge by developing the building blocks for a new family of foundation models based on knowledge graphs. To this end, we develop new approaches for the large-scale integration and embedding of knowledge graphs. We exploit these resources to develop hybrid explainable machine learning approaches that will later underpin our foundation model dubbed WHALE. In this short talk, we give a brief overview of our approach and first results.
Prof. Dr. Axel-Cyrille Ngonga Ngomo
Prof. Dr. Ngonga studied Computer Science and Physics at Leipzig University. In his doctoral studies, he developed unsupervised and weakly supervised methods for the extraction of ontologies from large text corpora. His work was granted the best student paper award at CICLing 2008. His habilitation focused on machine learning and rapid execution approaches for data integration. He then led the Agile Knowledge Engineering and Semantic Web group at Leipzig University, where he performed research on various topics related to the lifecycle of knowledge graphs. Since 2017, he is a full professor (W3) of Data Science at Paderborn University, where he is also the director of the Joint Artificial Intelligence Institute and of the Computer Science Computing Facility. He has received over 30 international awards for works on knowledge extraction, veracity, benchmarking, storage, and machine learning on knowledge graphs. Amongst others, he is one of the first two Lamarr fellows. Prof. Ngonga is currently a PI in over 15 national and international research projects and the coordinator of 2 international training networks.