Implicit Neural Image Representations and Inverse Problems
Day 2 | 11:00 – 12:00 | Main Hall
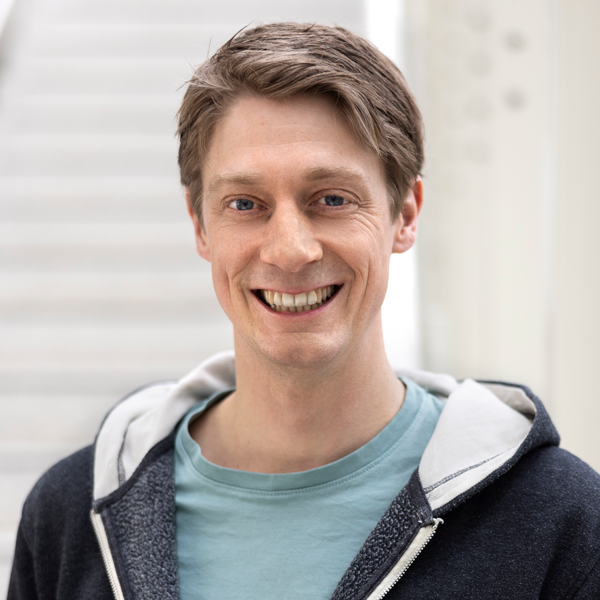
Prof. Dr. Michael Möller
University of Siegen
Abstract
In this spotlight talk, I will introduce the concept of implicit neural image representations, i.e., a powerful new paradigm of representing images as a function parameterized via a neural network. I will discuss why such representations are highly promising and which challenges the machine learning community faces in their use for inverse problems in imaging.
Prof. Dr. Michael Möller
Michael Möller studied and completed his doctorate in applied mathematics in applied mathematics in Münster from 2004 to 2012, during which time he spent two years as a researcher at the University of California, Los Angeles, UCLA during this time. After completing his doctorate with his supervisor Martin Burger, he worked for 1.5 years in the research and development department department of Arnold & Richter Cine Technik GmbH (ARRI) before joining the computer vision group of Daniel Cremers at the Technical Cremers’ computer vision group at the Technical University of Munich. Since 2016 professor at the University of Siegen. His main field of research is the combination of model- and learning-based methods for image reconstruction and analysis. He is part of the DFG priority program “Theoretical Foundations of Deep Learning”, spokesperson of the DFG research group “Learning to Sense” and was awarded a Lamarr Fellow in 2023.