Developing Model-Aligned and Human-Readable Explanations for Artificial Intelligence in Applications
Day 2 | 16:10 – 17:15 | Workshop Room 2
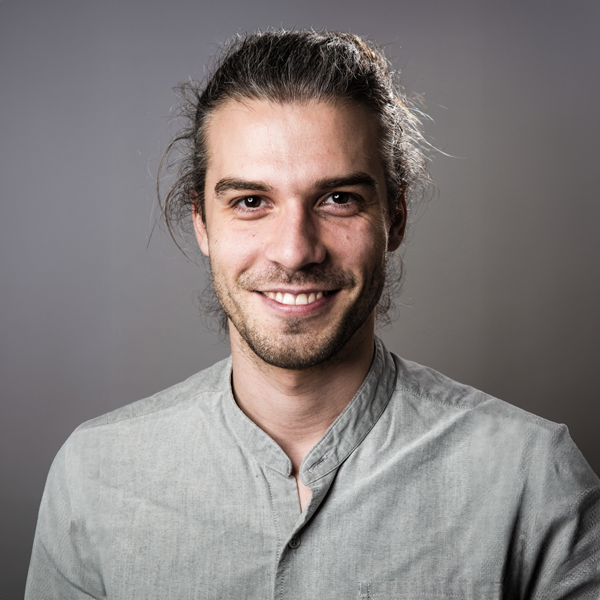
Thomas Schnake
TU Berlin, BIFOLD
Abstract
Explainable artificial intelligence (XAI) is increasingly critical for the applicability of AI, especially with the upcoming EU AI regulation (AI Act). However, the interpretation of machine learning models can vary significantly depending on the data and domain. In many applications, such as predicting properties in quantum chemistry and in natural language processing, classical explanations methods are not sufficient to enhance the analyst’s understanding. This talk explores how explanation methods can recover the complex decision-making processes of AI models and adapt them for human-readability, with a focus on different application domains.
Thomas Schnake
I’m a PhD student at the Technical University of Berlin and BIFOLD, with a background in mathematics (B.Sc. & M.Sc.) and scientific computing (M.Sc.). My research focuses on developing model-aligned, human-readable explainable AI solutions, particularly in quantum chemistry and natural language processing. I’ve had the opportunity to publish on these topics, including a journal paper in IEEE TPAMI and two ICML papers between 2021 and today.