Demystifying Graph Neural Networks in Drug Discovery via Explainable Artificial Intelligence
Day 2 | 12:30 – 13:00 | Workshop Room 3
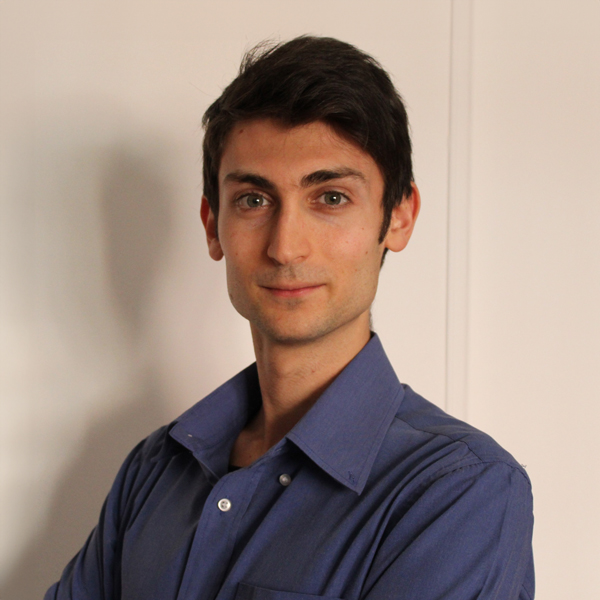
Dr. Andrea Mastropietro
Sapienza University of Rome
Abstract
Deep learning is a powerful tool for biomedical and life sciences applications, including drug discovery. Neural networks trained on huge amounts of data can deliver extremely accurate predictions. Yet, the opacity of these models and the impossibility to directly interpret their predictions work against the accessibility and trustworthiness of deep learning results . For this reason, explainable artificial intelligence (XAI) approaches can be developed to shed light on their predictions. Recently, graph neural networks (GNNs) have increasingly been used to learn from network-like data. In this context, the contribution will show how XAI can help to rationalize GNN-based compound activity predictions and unveil their learning characteristics when trained on protein-ligand interaction graphs, addressing a key question: Do GNNs really learn interactions or simply memorize data?
Dr. Andrea Mastropietro
Dr. Andrea Mastropietro is a Computer Engineer with a Ph.D. in Data Science and Postdoctoral Researcher at the Department of Computer, Control and Management Engineering (DIAG) of Sapienza University of Rome. Currenlty, he is a Visiting Postdoctoral Researcher at the Lamarr Institute at the University of Bonn. His research interests are in the field of AI for medicine, mainly focusing on deep learning and XAI in bioinformatics and chemoinformatics.